In the dynamic landscape of the financial sector, our recent project is looking to innovate with cutting-edge technology. We have engineered a robust pipeline for a forward-thinking startup, utilizing Natural Language Processing (NLP) and Knowledge Graphs (KG) to identify potential instances of fraud. At its core, our project involves the construction of a comprehensive KG of individuals and their relationships, sourced from diverse multilingual information news streams.
The primary challenge we addressed was to create a KG of individuals and their interconnections by intelligently parsing information from various sources, transcending language barriers. Leveraging entity-relation models, we successfully extracted essential data about individuals and their affiliations. This KG is hosted in Neo4j, providing a structured repository of knowledge.
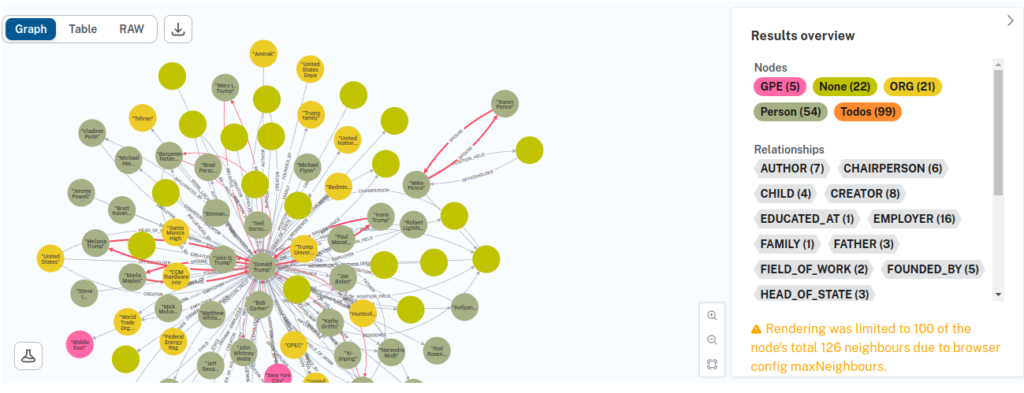
What sets our project apart is the use of a semantic search model, which we constructed using vectors derived from the KG. This model plays a pivotal role in validating relationships and disambiguating individuals. It empowers our system to not only identify connections but also to ascertain their validity, thereby strengthening the fraud detection process. To further validate relationships and disambiguate individuals, we harnessed the vast knowledge base of Wikimedia.
Our project exemplifies the extraordinary potential of NLP and KGs in enhancing security measures within the financial sector. It showcases how advanced technology can create a robust defense against fraudulent activities while seamlessly traversing linguistic and relational complexities.